HOW MIGHT WE:
Use Audio Transcription to help doctors complete their medical notes through the aggregation & display of relevant information.
Ultimate Goal:
Remove the Computer from the Consultation Room all together and create a more holistic experience for both Doctor and patient alike
Limitations:
1. Can AI accurately understand what information is relevant for the doctor during the consultation 2. Will such a system make healthcare professionals lazy and complacent? 3. How much information can be displayed on such small screens, without removing critical info?
Understanding the problem at hand
Electronic Medical Records - A brief history
Things have come a long way since the early days of medicine with a long list of achievements, inventions, and innovations. However, at the core of all of this progression is the notion of doctors cumulatively building their knowledge by sharing stories of ailments, treatments and findings from experiments.
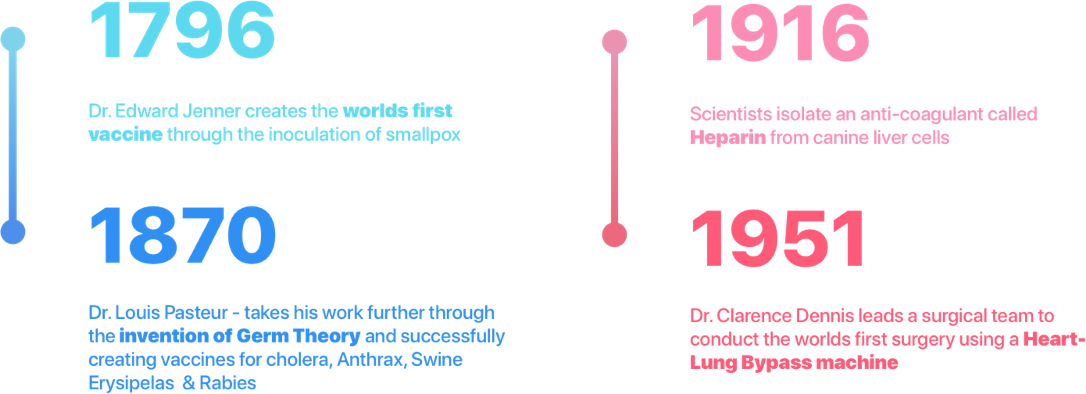
However, as more and more tasks such as creating and sending prescriptions, viewing and ordering blood (or other) tests, referrals, scheduling surgery, looking up past notes, hospital records, and billing the patient were added, doctors came to despise EMR systems and their computers all together.
In fact, a 2016 study conducted by the University of Wisconsin found that physicians were spending upwards of 2 hours for every 1 spent with the patient, irregardless of the brand or type of software used. This combined with the average work day of physicians increasing to 11 and a half hours has lead to severe burnout for doctors and some could say is one of the leading factors to doctor-patient relationship becoming ever more impersonal and scary. Moreover, in an attempt to save time (and increase efficiency), most clinics have relegated the first step to the nurse during triage or a preliminary examination and questionnaire. This has lead to a scenario where many patients aren't comfortable sharing the true intention of their visit until they see the doctor and one where the first half of the consultation is taken up by the physician staring at their screen and clicking through options.
As these needs increased many of the simple EMR programs had to be upgraded and in 2010 the first Electronic Health Record (EHR) systems came to fruition. These often virtualized systems attempted to answer healthcare professionals’ biggest dream of having a single connected database to access all patient files in one location. Much of this disparate data was made up of inconsistent recording methodology between organizations and locations, data stored in different physical locations, utilizing different data structures as well as many choosing to stick to old paper charts and notes for specific tests, as a first round of write up or throughout the facility.
As a means to curb these practises, new guidelines were by created by the Centres for Medicare and Medicaid (CMS) introducing improved diagnosis code system used to standardize all data entry for symptoms and diagnoses as the ICD9 code system (now updated to ICD10) in 2011 and by 2015 penalties were placed on any healthcare provider not using an accredited and compliant system.
Moreover, as technology and more importantly research into Machine Learning and Artificial Intelligence increased this vast amount of data became the centre of attention. Specifically, there has been a lot of hype around the combination of big data and deep learning to accurately detect specific types of aggressive tumours from MRI scans, enhance MRI, X-RAY, ultrasound and CT scan images, mapping of patient trajectory through the healthcare system and combining all patient data to begin to extract patterns & model the average population, as well as into segmented demographic models for a more accurate understanding of what is unhealthy.
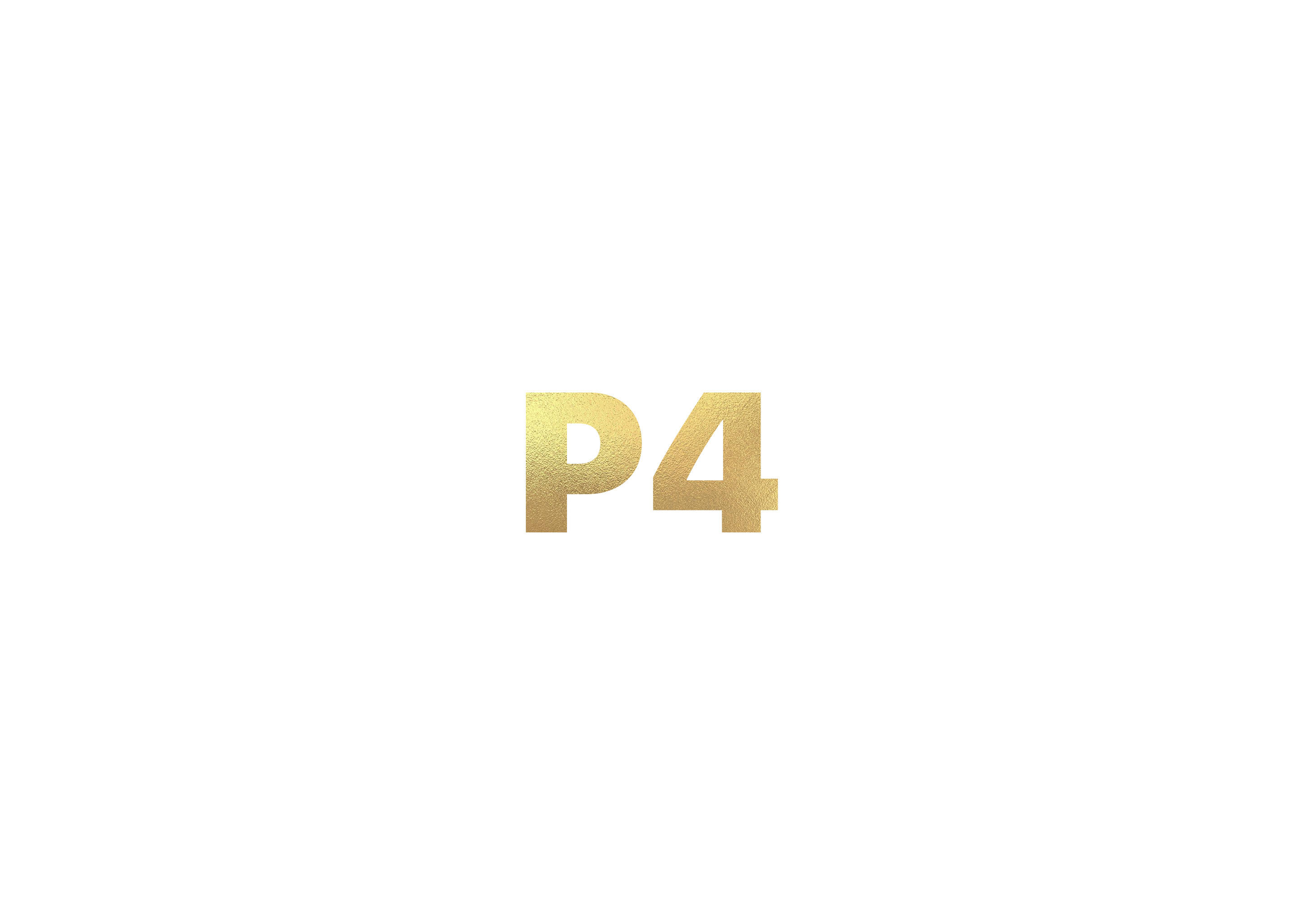
Unfortunately, the belief that throwing sufficient data and compute power at the problem will magically uncover these patterns is false. Instead current data sets and data collection methodologies are best suited to modelling binary outputs - does this image contain a tumour, is this patient undergoing a hidden and early atrial fibrillation or slightly more complex predictions based on non-linear calculations such as the DAS28 test used to classify Rheumatoid Arthritis into 3 categories (low, medium, high).
Moreover, such a focus on comparing an individuals health to the average populous or demographic ignores moves us away from the golden ideal of healthcare encompassed by stratified medicine . This approach also known as p4, personalised and precision medicine is a process by which patients are grouped by their disease subtype, risk, prognosis, or treatment response using specific and customized diagnostic tests. The main idea here is to first understand an individual patients’ characteristics (including molecular, behavioural, environmental and other biomarkers) and by using this as a baseline to define “healthy” on a per person basis, accommodate for the ebbs and flows of reality and help reduce the ambiguity in medicine.
This goal is currently blocked due to an inadequate number of clinical endpoints, insufficient study validation, lack of sufficient mechanistic modelling and causal understanding of observed phenomena.
Better Understanding the User
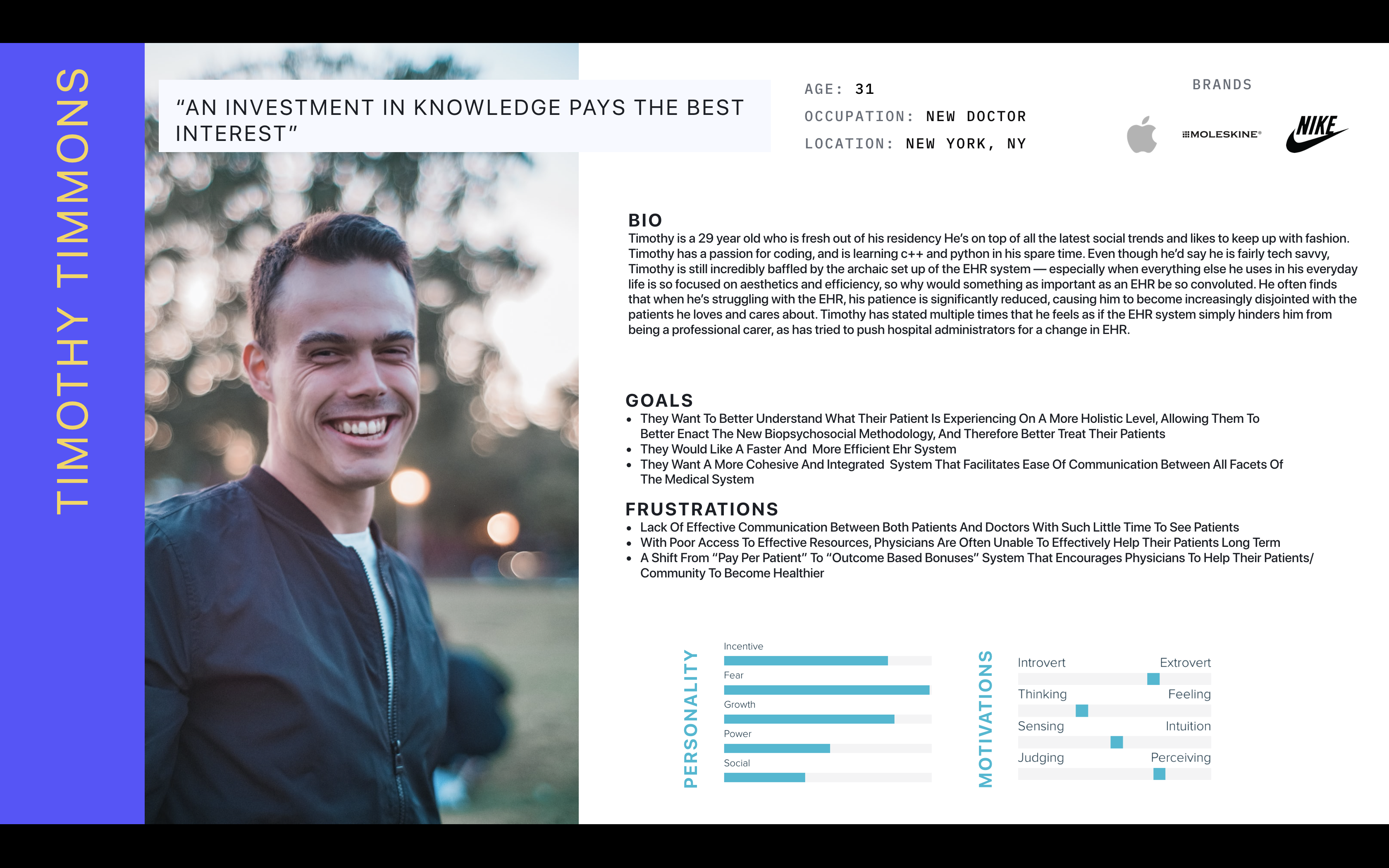
Dr. Tim’s Current Task Flow
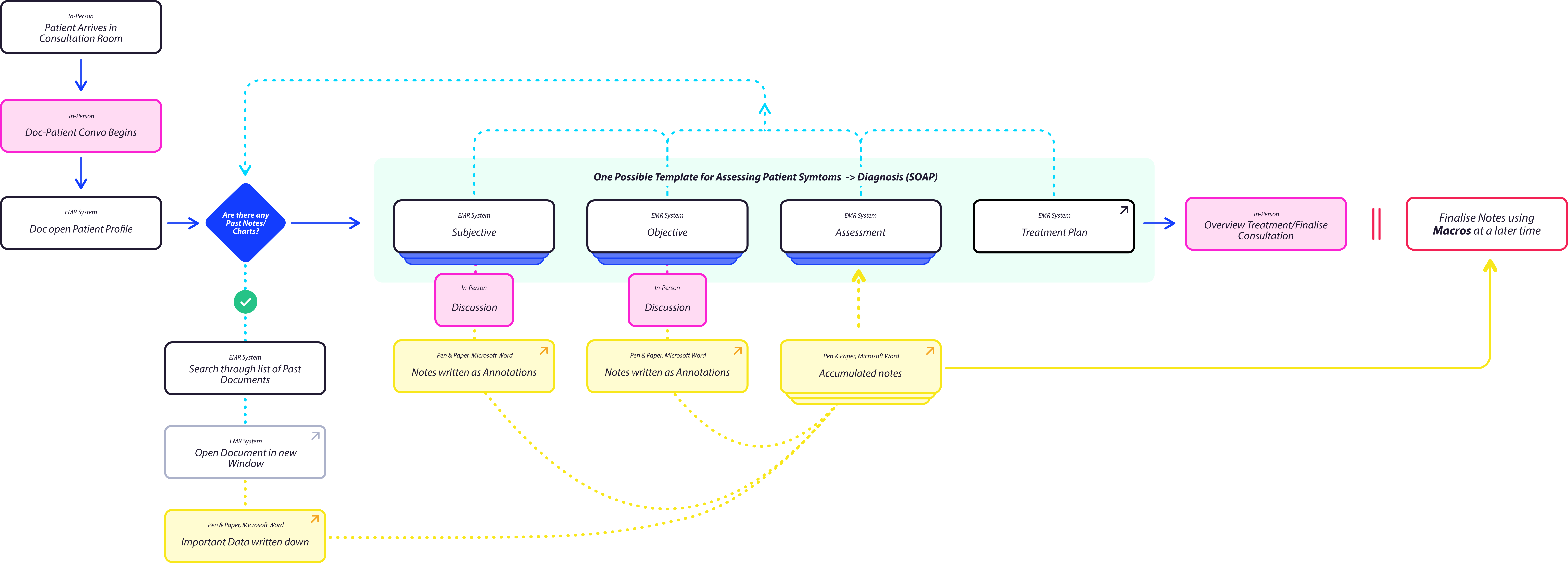
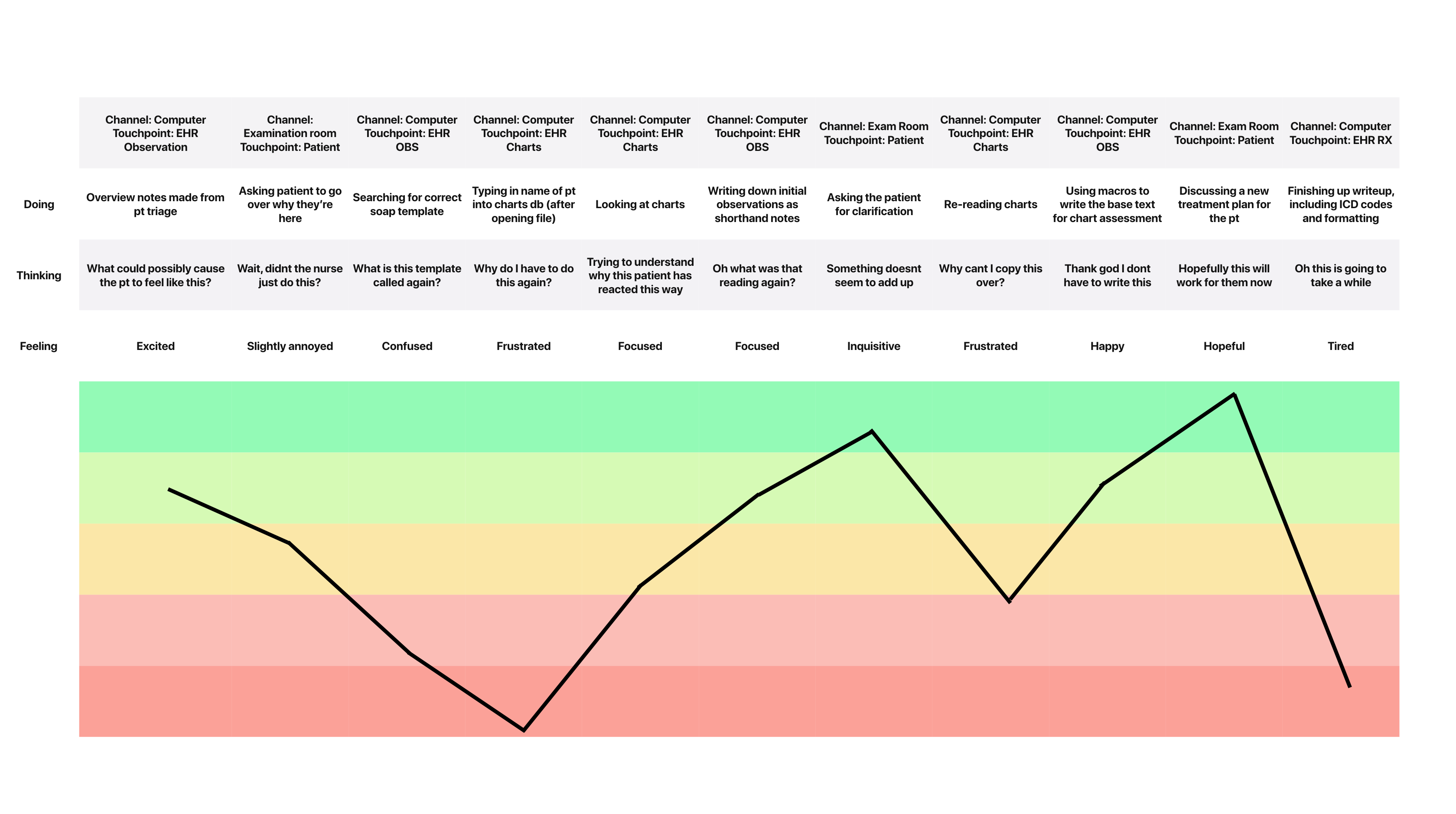
Key Insights
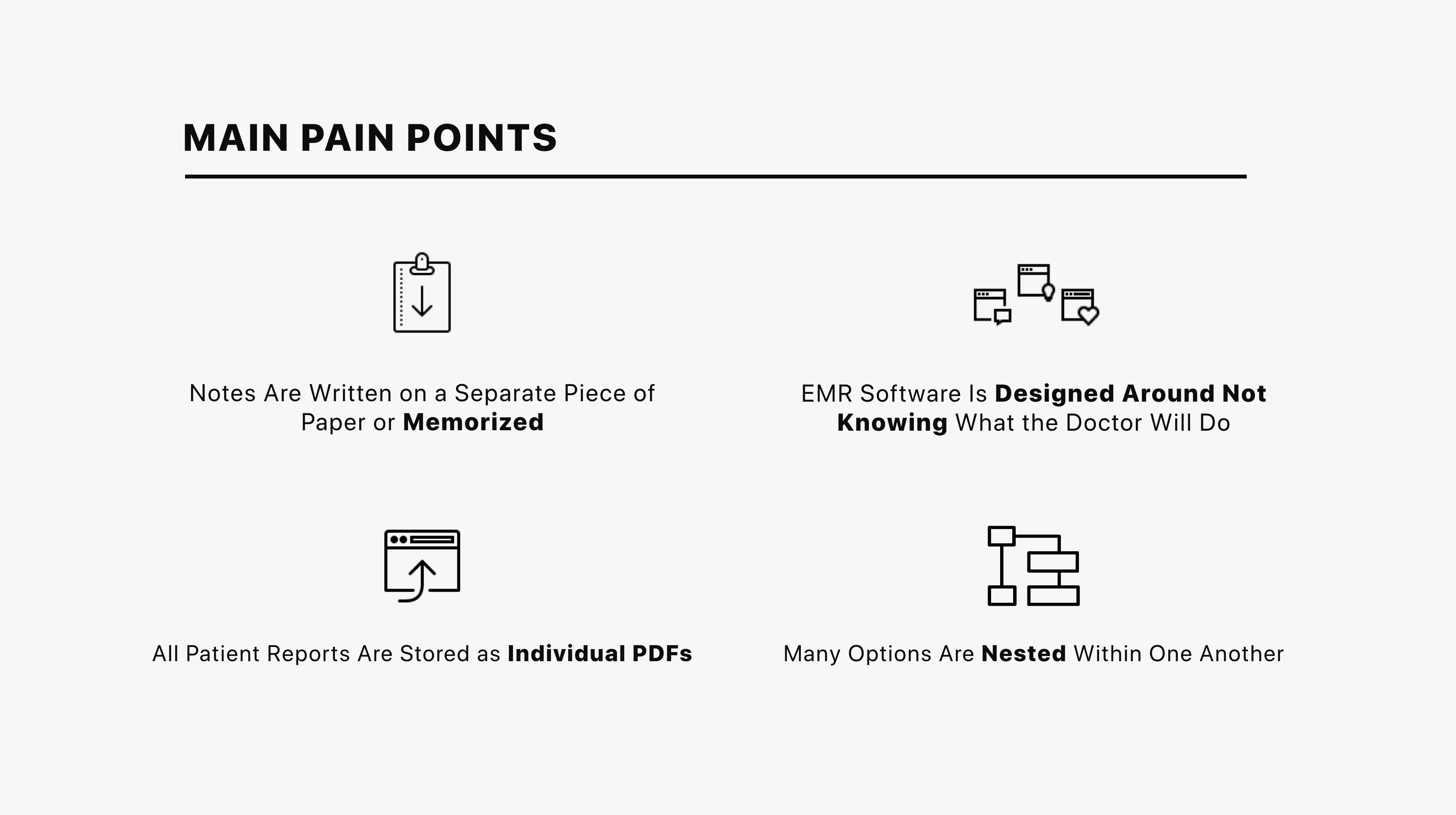
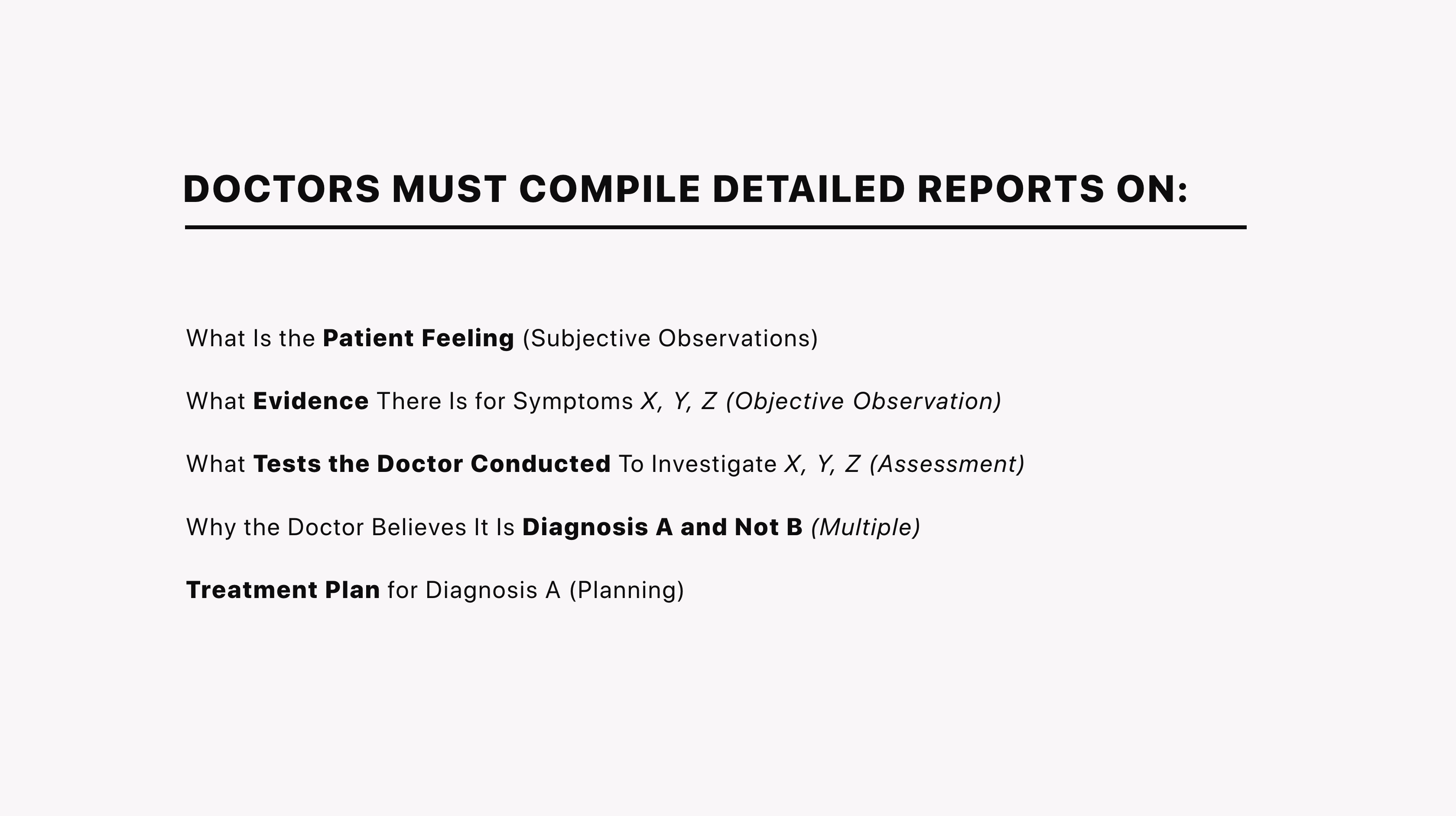
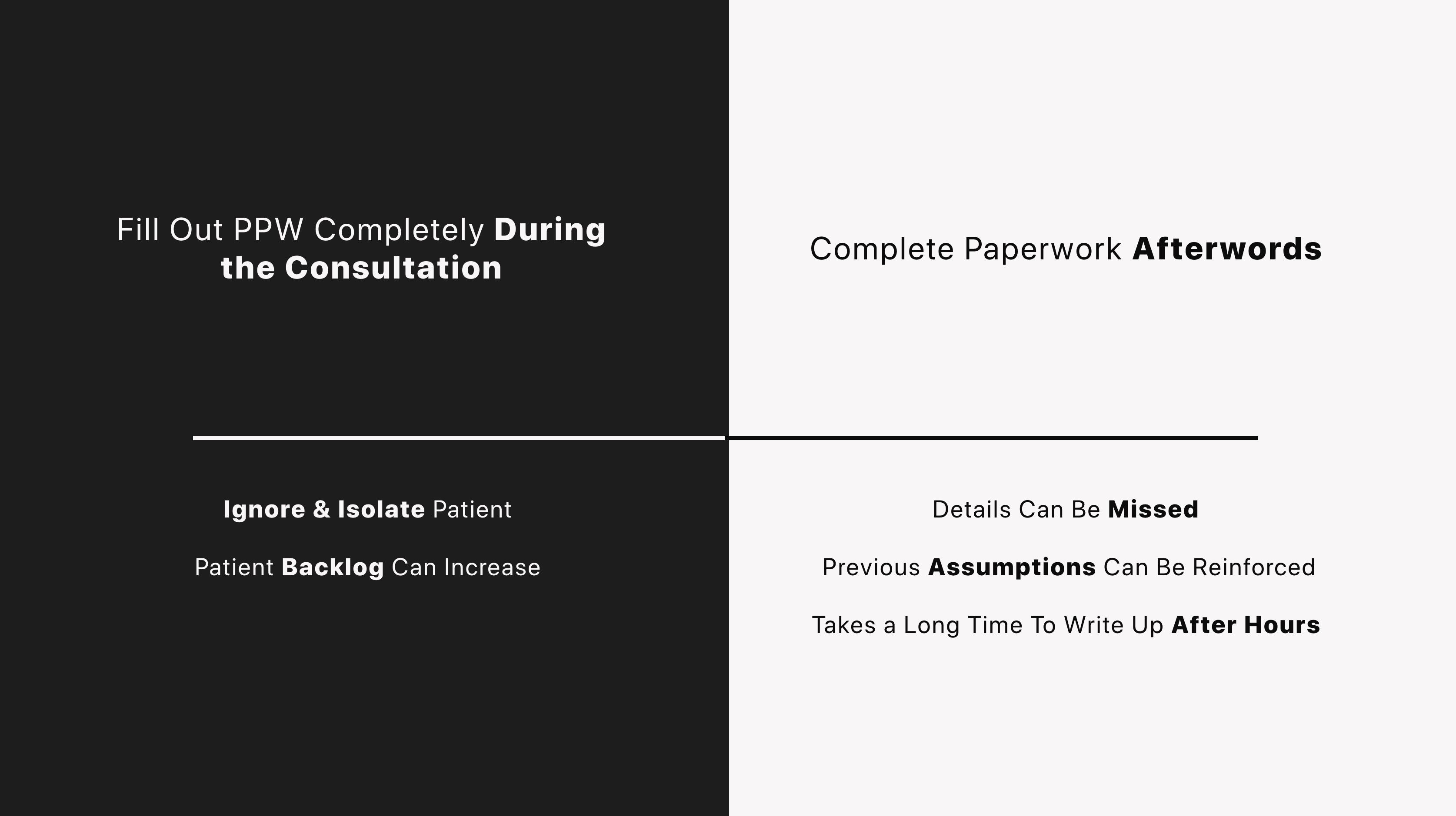
Exploring and Reshaping the User Flow
Before we actually jump into the user flow, I feel it is important to overview why we can’t simply transcribe a word directly to a diagnostic flag but overally the problem revolves around the fact that in each phrase is hidden context, which can easily be lost, misrepresented in the data or simply switched between flags. For example the following phrase can be broken down into many categories such as Age and Currently Existing Conditions, which all lead to their own path of specific diagnosis.
![]()
Version 1 - After Consultation Report
When designing my first solution it became clear to me that we needed to decrease the overall number of options presented to the doctor at any given time. To do this I implemented a two layer filtering system, where the intitial symptoms taken from the patients’ Reason for Visit are used to find Recommended Templates
![]()
Invision Link
![]()
![]()
Version 2 - Adaptive Templates
![]()
Version 3 - Shifting Focus
![]()
Design changes
Wrap up
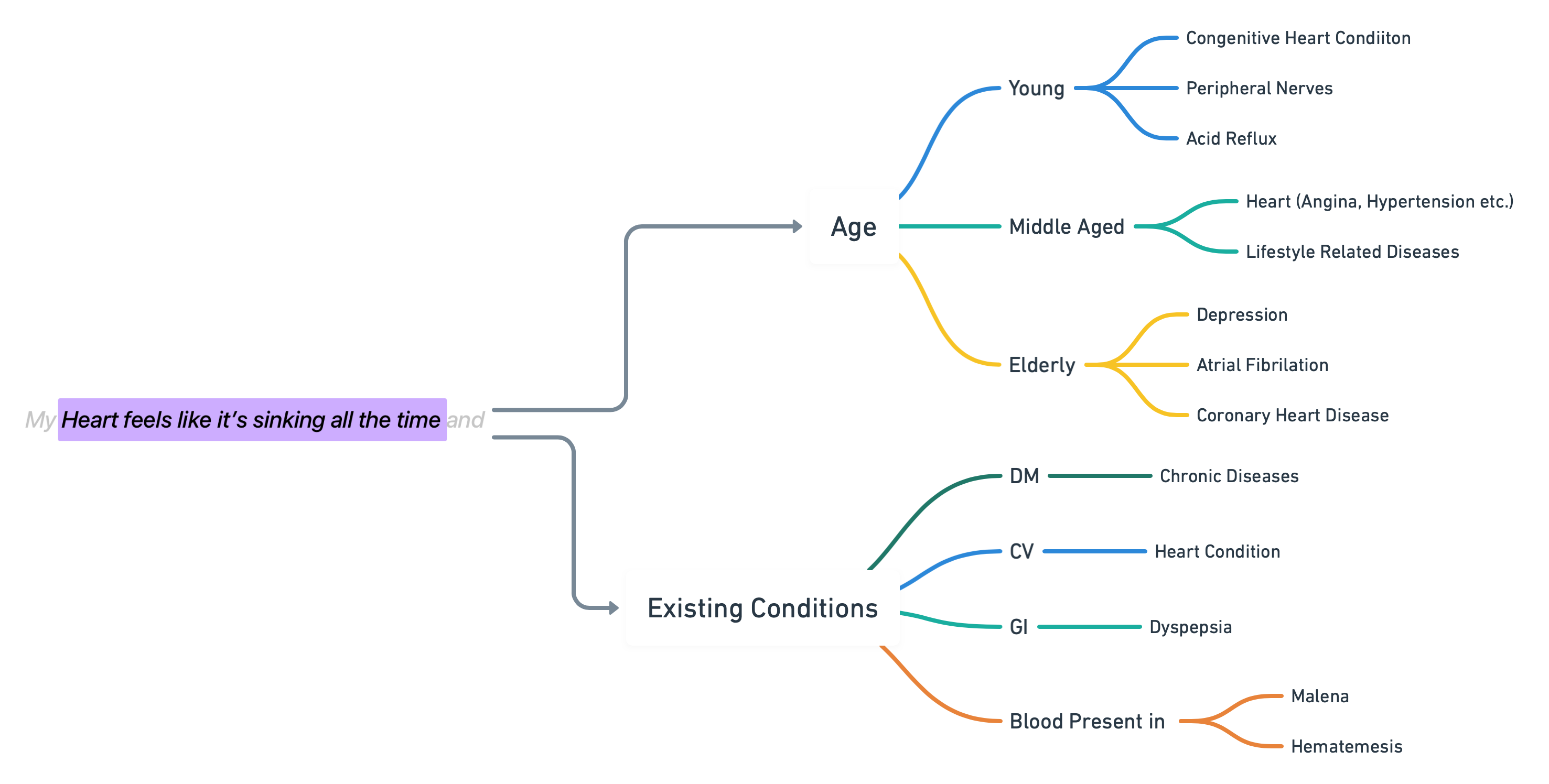
Version 1 - After Consultation Report
When designing my first solution it became clear to me that we needed to decrease the overall number of options presented to the doctor at any given time. To do this I implemented a two layer filtering system, where the intitial symptoms taken from the patients’ Reason for Visit are used to find Recommended Templates
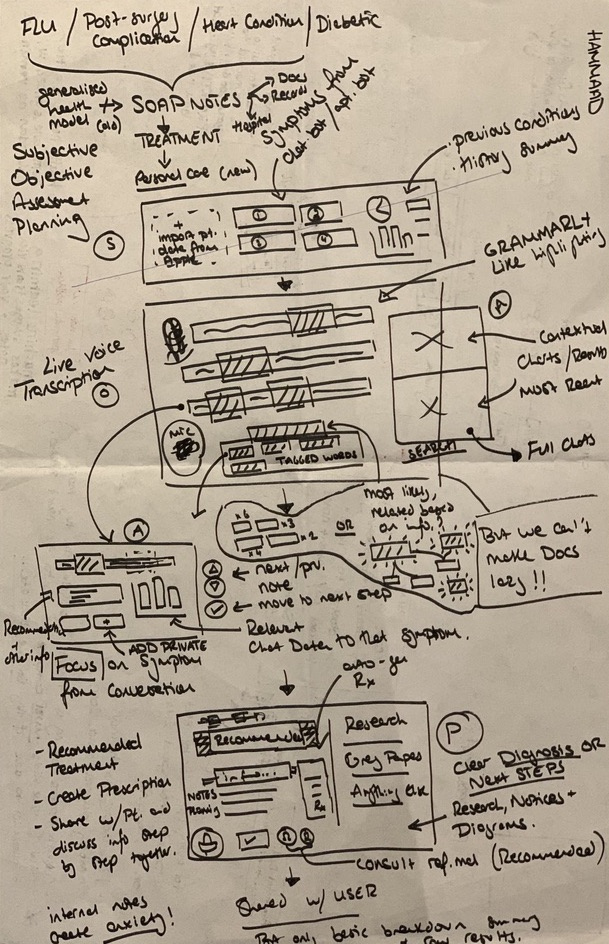
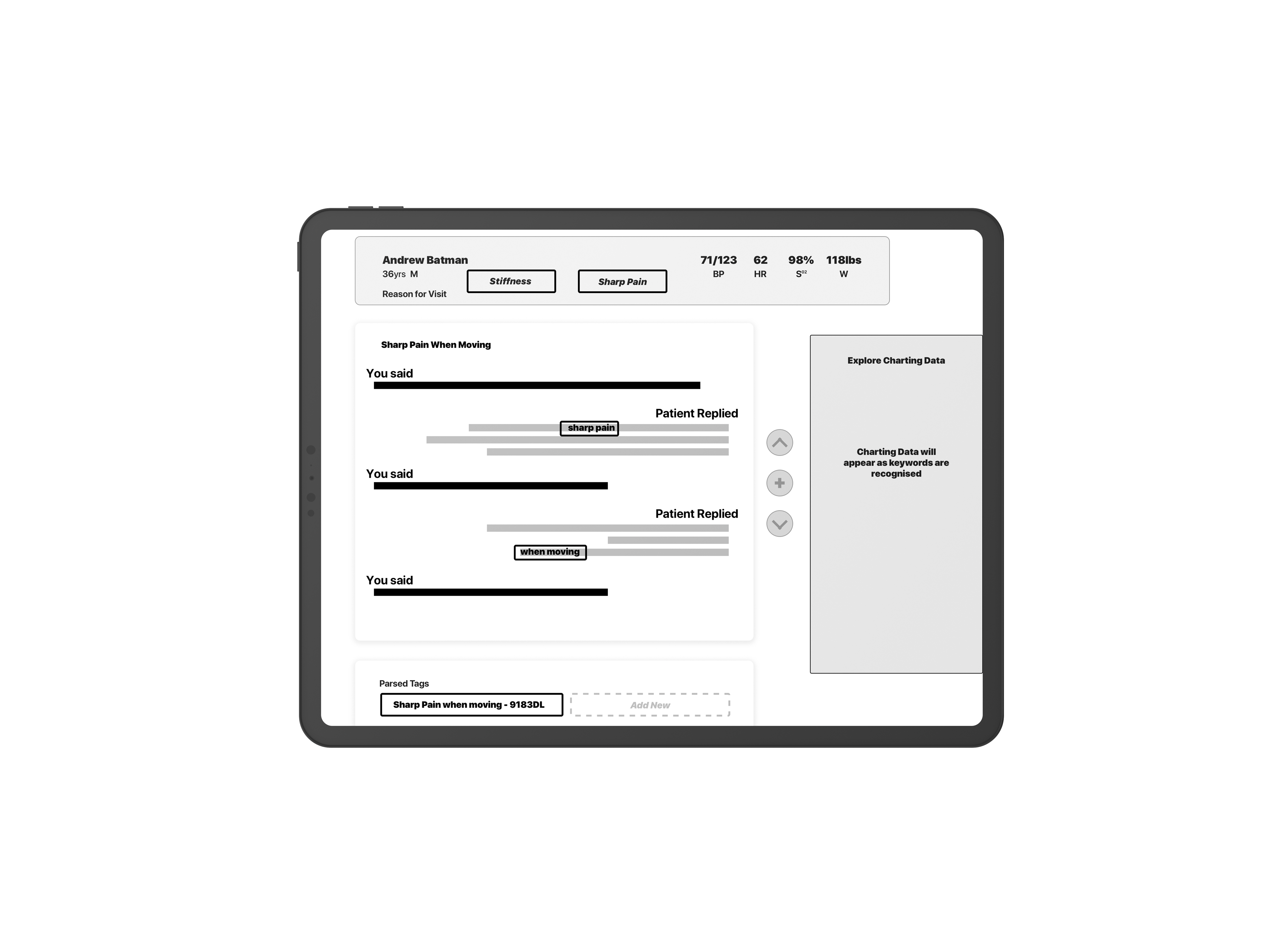
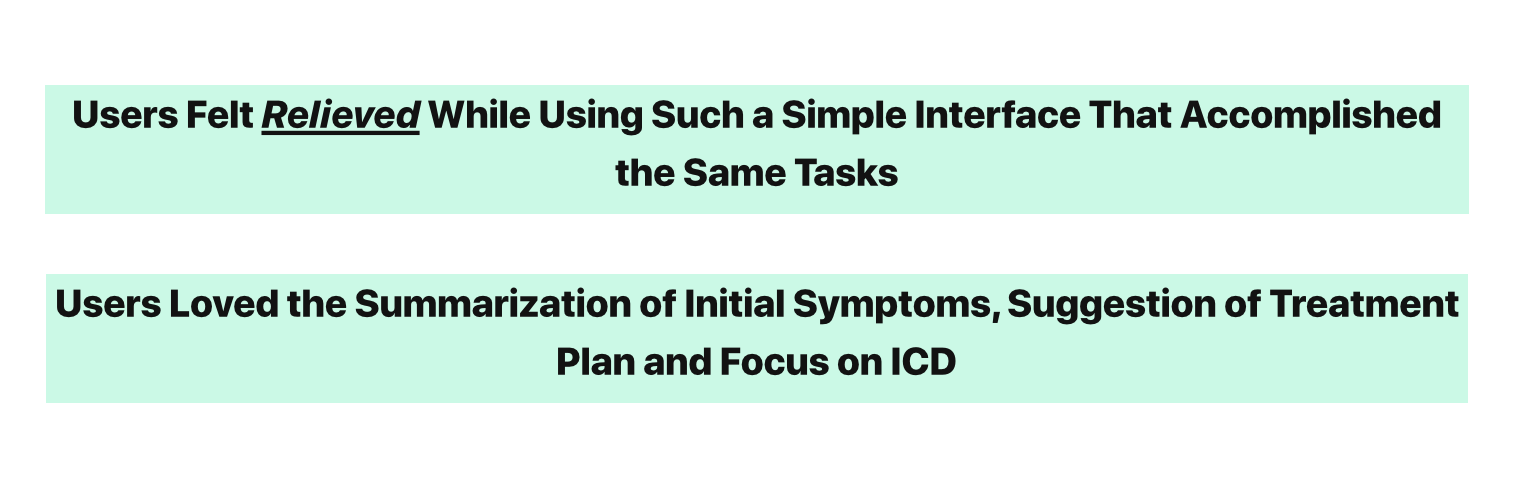
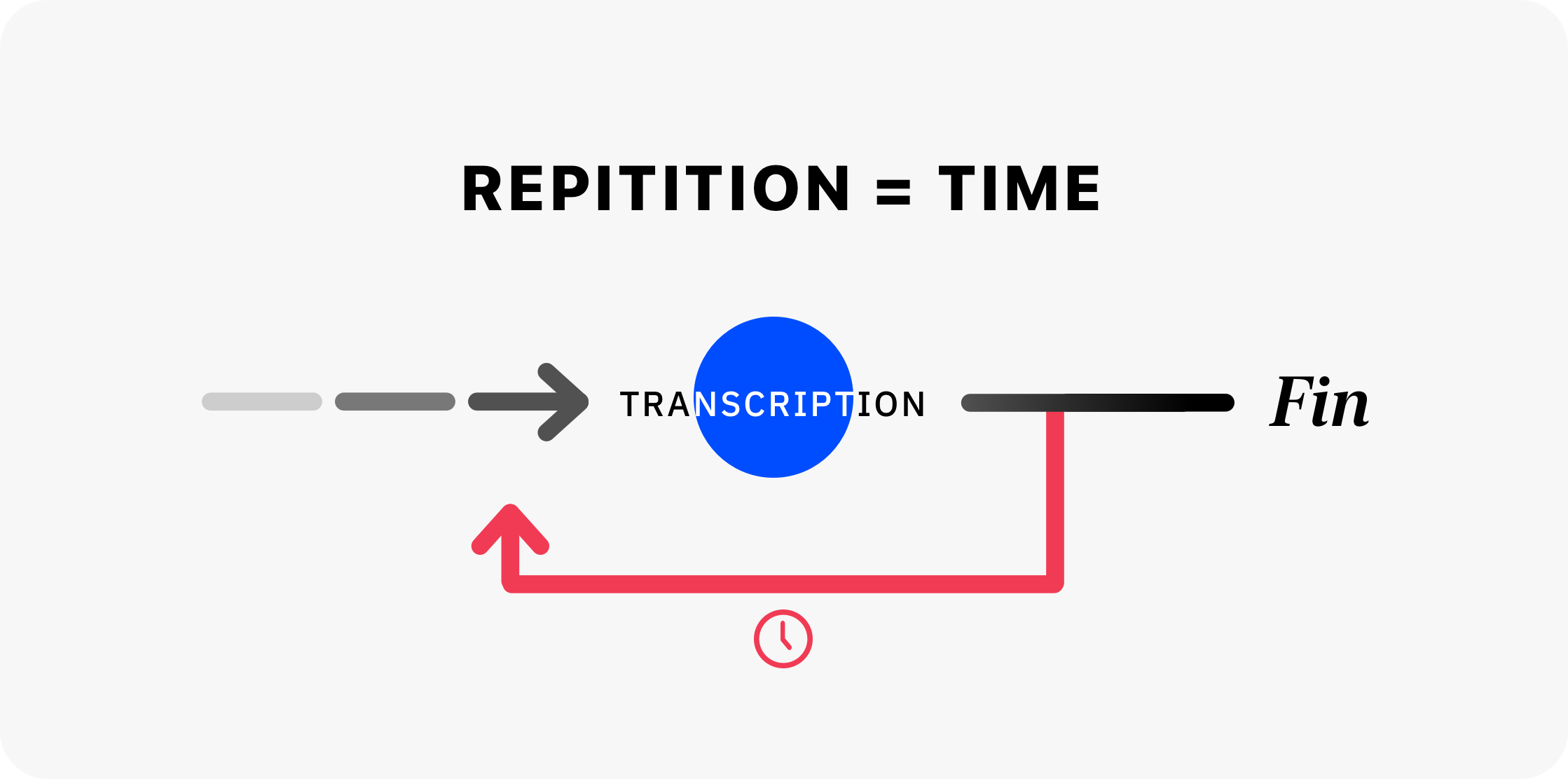
Version 2 - Adaptive Templates
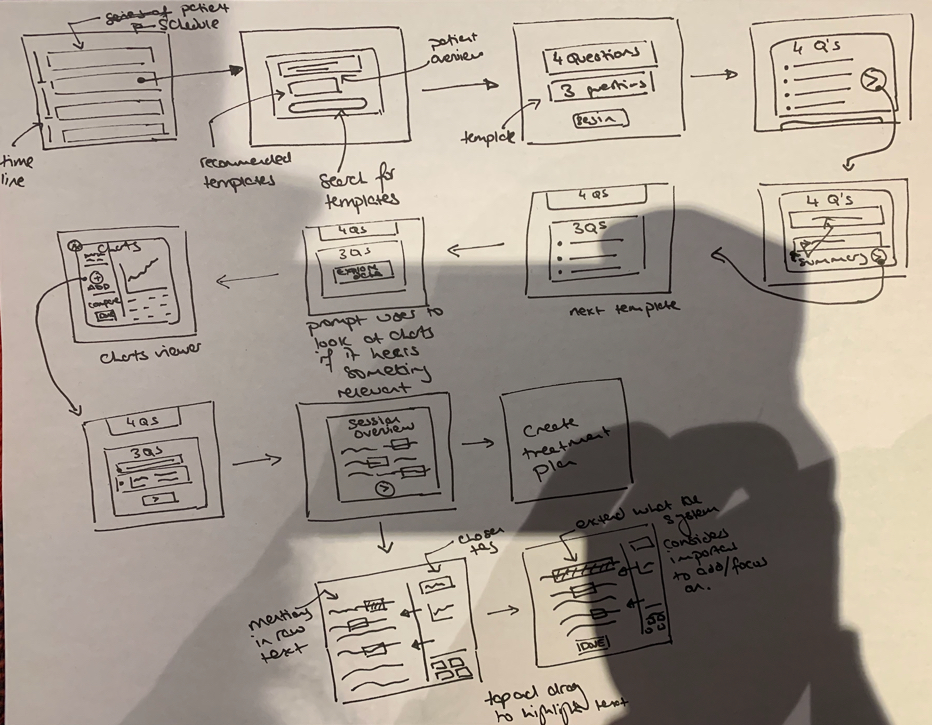
Version 3 - Shifting Focus

Design changes
Wrap up